
Our Objective
By April, 2020
It’s estimated that every year about 7.9 million infants (6 percent of worldwide births) are born with serious birth defects, about 3% of those defects are facial deformations. In order to overcome such deformities, reconstruction surgeries are required. However, reconstruction surgeries can be a challenge for everyone, whether it’s the patient, patient’s family, or even the surgeon himself. According to surveys and interviews conducted by the team, both the patients and the patients’ families consider such surgeries to be extremely risky and not always reliable, especially that the reconstruction area would be the face, making any mistake ruinous. Meanwhile surgeons find it difficult to generate faces of their patients without any deformations. Currently, in order to create a generate face, doctors need to use morphing tools, such as photoshop, in order to create the most normalized image of the patient without any facial deformations. This generated image is then used as a reference for the surgeon to proceed with the surgery procedure. However, such a task requires a thorough and detailed imagination to master all the features required to normalize the face, and that always arises a risk of inaccuracy due to either lack of imagination, or personal beauty standard bias that might unintentionally affect the results of the generated image. To help overcome this dilemma, a software by the name of the Facial Generation and Anomaly Detection Software is currently being built by the team. The software uses generative adversarial networks (GANs) in order to generate the most accurate, normalized image of the patient without any facial deformations. This method (GANs) consists of pitting two neural networks against each other in a game (often more than not a zero-sum game), hence the word “adversarial”. GANs are self-supervised and self-taught systems, that means that GANs automatically figure out the patterns, similarities, and differences in input data after a while of practice. That helps the GANs generate very accurate models and examples of expected outcomes that are based on the original input. Moreover, the application will also be embedded with a scale that measures the success rate of the surgery. This is executed by inserting the picture of the patient post-surgery, and using support vector machine (SVM) and local outlier factor (LOF) methods, the applications will compare the inserted picture to the generated picture (used as a reference), and give us a numerical value representing the rate of similarity, hence determining the rate of improvement. The application is built with the hope that with its presence, the patients would go into such surgeries with their minds at ease especially that reconstruction surgeons would hopefully be able to achieve more accurate results since they will be provided with more accurately generated normalized reference pictures.
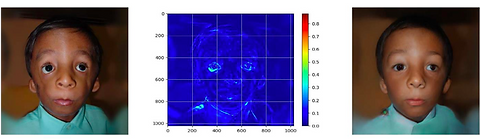